Scientists have harnessed the power of artificial intelligence (AI) to develop a groundbreaking predictive model for identifying the most potent cancer-killing immune cells for personalized cancer immunotherapy. This innovation holds immense promise for tailoring treatments to the unique characteristics of each patient’s tumour (Figure 1).
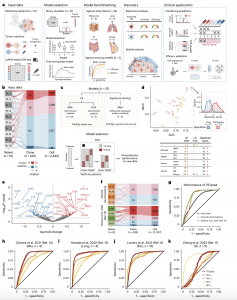
Figure 1: TRTpred, a sensitive in silico predictor of tumour-reactive clonotypes. a, Illustration of TRTpred design, benchmarking and applications. The final algorithm, MixTRTpred, combines TRTpred with a structural avidity predictor 16 and TCRpcDist18, a TCR clustering algorithm. b, Alluvial plot showing the fractions of cells and clones annotated as tumour-reactive or non-tumour-reactive (orphan or antigen (Ag)-specific) within the input data (n = 10 patients with melanoma). c, Top, design of the 12 LR and 9 signature scoring models with their hyperparameters (Methods). Bottom, model selection framework estimating the generalization performance of the model through an LOPO NCV. d, Evaluation of the 12 LR (yellow circles) and 9 signature scoring (pink triangles) binary classifiers in the function of MCC and the AUC (Supplementary Table 2). The panel shows the distribution of the best model scores for tumour antigen-specific (red) and viral-specific (blue) clones. e, Volcano plot displaying the differential gene expression analysis comparing tumour-reactive and non-tumour-reactive cells. The 90 upregulated (red) and downregulated (blue) genes obtained by edgeR-QFL are shown (Supplementary Table 4). The P values are calculated using the two-sided quasi-likelihood F-test in the edgeR package and are corrected for multiple testing using the Benjamini–Hochberg procedure. f, Alluvial plots showing the fractions of cells and clones annotated as tumour-reactive or non-tumour-reactive (orphan or Ag-specific) within internal (top) and external (bottom, ref. 14) benchmarking data. g, ROC curve of TRTpred applied on the input data (black), and the internal (orange) and external (green, ref. 14) benchmarking data. h–k, ROC curves of TRTpred and four CD8+ TIL tumour-reactive predictive signatures (refs. 8,11,12,14) applied to the four datasets: ref. 14 (melanoma, n = 4) (h), ref. 12 (lung, n = 4) (i), ref. 8 (n = 1 melanoma, n = 2 breast and n = 12 GI) (j) and ref. 11 (GI, n = 5) (k). All AUCs are reported in Extended Data Fig. 3e. Pt, patient; TAA, tumour-associated antigen; UMI, unique molecular identifier; PCA, principal component analysis; Mel, melanoma; Pan, pan-cancer.
Cellular immunotherapy is a promising cancer treatment that leverages a patient’s own immune system. T cells, a type of white blood cell, play a crucial role in recognizing and eliminating abnormal cells. This therapy involves extracting T cells from a patient’s tumour, potentially enhancing their anti-cancer abilities, and reintroducing them to fight the cancer. However, not all T cells within a tumour are equally effective.
The research team developed TRTpred, a powerful AI model that ranks T cell receptors (TCRs) based on their ability to target and destroy cancer cells. This model can then be applied to analyze new T cell populations, with impressive accuracy. In a test involving 42 patients with various cancers, TRTpred successfully identified tumour-reactive TCRs with a 90% success rate.
To further refine the T cell selection process, the researchers implemented additional filters. One filter identifies T cells with high avidity, meaning they bind strongly to cancer antigens (targets) on tumour cells. They found that T cells flagged by TRTpred and this secondary filter were more likely to be located deep within tumours, indicating their ability to infiltrate and attack the cancer.
A final filter prioritizes diversity in the TCRs selected. The goal is to create a team of T cells that can recognize a broad range of cancer antigens. This final step groups TCRs based on similar characteristics, with the assumption that those within a group target the same antigen. This combined approach, utilizing TRTpred and all three filters, is called MixTRTpred.
To validate their approach, the researchers used MixTRTpred to identify potent T cells from human tumours grown in mice. These T cells were then reintroduced into the mice and demonstrated exceptional effectiveness in eliminating the tumours. This success in a preclinical model paves the way for further investigation and potential human trials.
The development of MixTRTpred represents a significant leap forward in personalized cancer immunotherapy. By harnessing AI for T cell selection, researchers can potentially create highly effective treatments tailored to each patient’s unique cancer profile. This innovation offers renewed hope for a future where immunotherapy can effectively combat a broader range of cancers.
Journal article: Pétremand, R., et al., 2024. Identification of clinically relevant T cell receptors for personalized T cell therapy using combinatorial algorithms. Nature Biotechnology.
Summary by Stefan Botha